Details
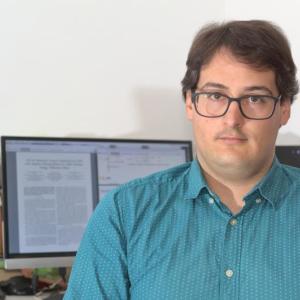
- Affiliation
-
AffiliationUniversidade de Santiago de Compostela
- Country
Neural network mixed-mode hardware accelerators for deep convolutional neural networks (CNN) strive to cope with a high number of input feature maps and increasing bit depths for both weights and inputs. As an example of this need, the ResNet model for image classification comprises 512 3 × 3 feature filters in its conv5 layer. This would lead to 4068 multipliers driving a summing node for actual concurrent processing of all the input feature maps, which makes up a challenge in mixed-mode. This paper addresses the design of a 5-bit signed SRAM-based in-memory computing cell in 180 nm 3.3 V CMOS technology, dealing with the impact of increasing the number of input feature maps. The data presented in the paper are based on electrical and post layout simulations.