Details
Presenter(s)
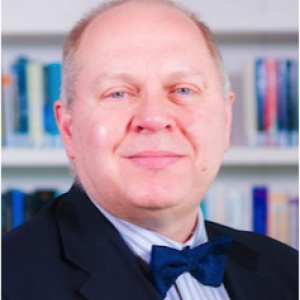
Display Name
Abe Elfadel
- Affiliation
-
AffiliationKhalifa University
- Country
Abstract
With the phenomenal growth of deep learning paradigms based on the use of the rectified linear unit (ReLU) as activation function and the importance attached to the hardware acceleration of such learning approaches, there is a pressing need for the development of numerical simulation algorithms that are tailored for the specific context of analog ReLU networks. In this paper, we propose two time-stepping schemes for the transient analysis of analog ReLU networks and provide rigorous proofs of their convergence under mild conditions on the ReLU network connectivity matrix. Simulation examples are provided that illustrate the numerical stability of these schemes and contrast their convergence rates.