Details
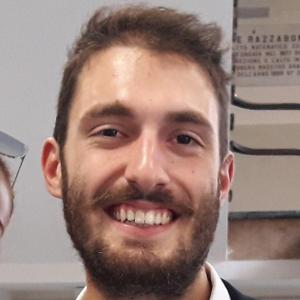
- Affiliation
-
AffiliationUniversità di Bologna
- Country
An important problem in magnetic resonance imag-ing (MRI) is the long time lapse required to acquire a fullysampled, high resolution scan. To speed up acquisition, Com-pressed Sensing (CS) has been used and recently coupled withNeural Networks (NN). In the latter setting, commonly CS hasbeen split into two different problems:i) design of the encoder,or selection of the undersampling pattern, andii) design ofthe decoder. A significant progress was recently introduced bya solution (called LOUPE) where encoding and decoding aresimultaneously addressed. Here we propose an improvementof this model, called ”regularized-LOUPE” (r-LOUPE), whichaddmeasurement constraintinto the picture, resulting in a×8speed-up in the MRI acquisition time. A further benefitof our methodology is that measurement constraint can beleveraged to implement a self-assessment tool able to predictthe reconstruction error and to identify possible out-layers.