Details
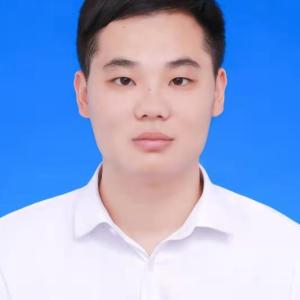
- Affiliation
-
AffiliationFudan University
- Country
-
CountryChina
Weakly supervised video anomaly detection (WS-VAD) aims to locate clips containing abnormal events in videos using the available video-level labels. In this paper, we introduce the thought of unsupervised video anomaly detection to the WS-VAD and propose a collaborative normality learning framework to explore task-specific representations for WS-VAD. Specifically, the auto-encoder is first trained in an unsupervised manner to learn the prototypical spatial-temporal patterns of normal videos. Then, the abnormal videos with video-level labels are used to train the channel attention-based regression module to calculate the anomaly score, where the goal is to make the average score of the abnormal videos higher than the maximum score of the normal videos. Finally, the clips in abnormal videos with a score lower than the average are fed into the auto-encoder to explore task-specific representations. Experimental results on standard benchmarks demonstrate that the proposed method outperforms the existing state-of-the-art methods, achieving the AUC of 83.1%, 95.5%, and 97.8% on the UCF-crime, ShanhaiTech weakly, and reorganized UCSD Ped2 datasets, respectively.