Details
Presenter(s)
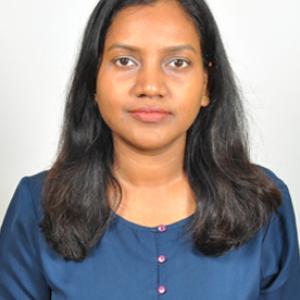
Display Name
Verma Pratibha
- Affiliation
-
AffiliationIndian Institute of Technology, Hyderabad
- Country
-
CountryIndia
Abstract
Traditional DNN compression is applicable during training to obtain an efficient inference engine. When the inference engine runs on the hardware platform, constrained by battery backup it brings additional challenge in terms of reducing the complexity (like memory requirement, area on hardware etc.). To reduce the memory complexity, we are proposing a new low complex methodology named as Clustering Algorithm to eliminate the redundancies present within the filter coefficients (i.e. weights). This algorithm is a three stage pipeline: quantization, coefficient clustering and code-assignment, that work together to reduce the memory storage of neural networks.