Details
Presenter(s)
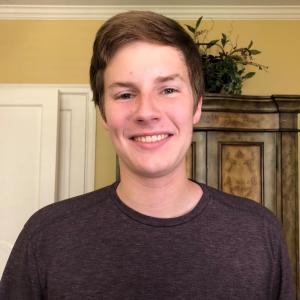
Display Name
Connor Talley
- Affiliation
-
AffiliationGeorgia Institute of Technology
- Country
Abstract
Compute in-memory (CIM) is an exciting circuit innovation that promises to increase effective memory bandwidth and perform computation on the bitlines of memory sub-arrays. Utilizing embedded non-volatile memories (eNVM) such as resistive random access memory (RRAM), various forms of neural networks can be implemented. Unfortunately, CIM faces new challenges traditional CMOS architectures have avoided. In this work, we characterize the impact of IR-drop and device variation (calibrated with measured data on foundry RRAM) and evaluate different approaches to write verify. Using various voltages or pulse widths we program cells to offset IR-drop and demonstrate a 136.4$\\times$ reduction in BER during CIM.