Details
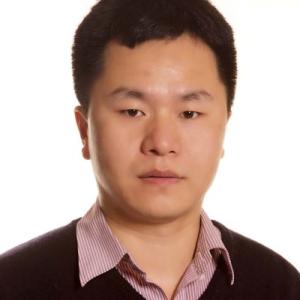
- Affiliation
-
AffiliationInstitute for Infocomm Research, Agency for Science, Technology and Research
- Country
-
CountrySingapore
Computationally intensive simulations have made analog circuit sizing challenging for complicated analog circuit performance characterization. Accurate yet computationally efficient data-driven models of circuit performance can potentially accelerate the design and verification process. However, as analog circuits are designed under strict functional and technology constraints, there is a scarcity of data for analog circuit performance classification, posing challenges to data-driven approaches; acquiring more data typically involves running expensive and time consuming simulations. We propose Bayesian Deep Active Learning (BDAL) to learn models using less simulations, by iteratively selecting a small number of informative samples to label based on the model uncertainty. Compared with the state-of-the-art approaches, the proposed BDAL method can obtain better classification performance with much less number of simulations. Experiments on four diverse analog circuits demonstrate BDAL can achieve significant reduction in data requirement and obtain similar performance with much fewer labeled data for analog circuit performance classification.