Details
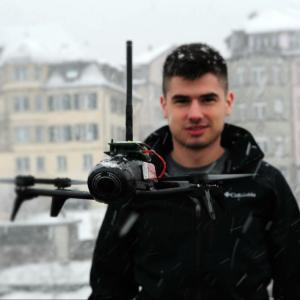
- Affiliation
-
AffiliationETH Zürich
- Country
-
CountrySwitzerland
Convolutional neural networks (CNNs) are fueling the advancement of autonomous palm-sized drones, i.e., nano-drones, despite their limited power envelope and onboard processing capabilities. Computationally lighter than traditional geometrical approaches, CNNs are the ideal candidate for directing inference from the sensor inputs. However, these sophisticated CNNs require significant complexity reduction to be deployed aboard a flying nano-drone. To date, these optimizations are mostly hand-crafted and require labor-intensive iterative development flows. In this work, we discuss methodologies and software tools to automate all the deployment stages on a low-power commercial multicore System-on-Chip (SoC), investigating an industrial and an academic tool-set.