Details
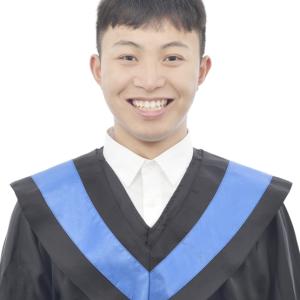
- Affiliation
-
AffiliationGraduate Institute of Electronics Engineering, National Taiwan University
- Country
Computing-in-memory (CIM) has demonstrated the great potential of analog computing in improving the energy efficiency of matrix-vector multiplications for deep learning applications. Albeit low-power feature of CIM, the non-linearity of digital-to-analog converters (DACs)/analog-to-digital converters (ADCs) causes deviation between the computed outputs and desired values, thus degrading classification accuracy. This paper proposes Automated Quantization Range Mapping (A-QRM) mechanism to mitigate the negative effect of non-linearity on model accuracy. Instead of fixing the quantization range for quantized deep learning models, the proposed A-QRM automatically finds a better quantization range that balances the model capability and quantization errors caused by the non-linearity. Experimental results show that our proposed A-QRM achieves 89.02% and 84.75% of top-1 accuracy in ResNet20 and VGG8 on Cifar-10, respectively, under the non-linearity of DACs/ADCs.