Details
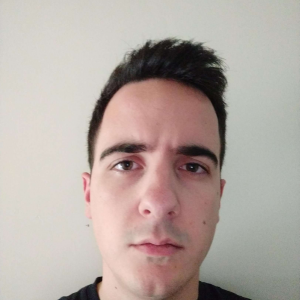
- Affiliation
-
AffiliationKing Abdullah University of Science and Technology
- Country
Speaker diarization is a task to identify “who spoke when”. Moreover, nowadays, speakers’ audio clips usually are accompanied by visual information. Thus, in the latest works, speaker diarization systems performance has been improved substantially by taking advantage of the visual information synchronized with audio clips in Audio-Visual (AV) content. This paper presents a deep learning architecture to implement an AV speaker diarization system emphasizing Voice Activity Detection (VAD). Traditional AV speaker diarization systems use hand-crafted features, like Mel-frequency cepstral coefficients, to perform VAD. On the other hand, the VAD module in our proposed system employs Convolutional Neural Networks to learn and extract features from the audio waveforms directly. Experimental Results on the AMI Meeting Corpus indicated that the proposed multimodal speaker diarization system reaches a state-of-the-art VAD False Alarm rate due to the CNN-based VAD, which in turn boosts the whole system’s performance.