Details
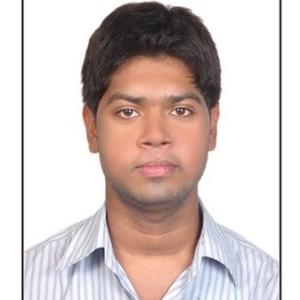
- Affiliation
-
AffiliationIndian Institute of Technology Hyderabad
- Country
In this paper, we propose two models to predict 26 parameters data of post clock tree synthesis (post-CTS) quality of results (QoR) report without running the CTS optimization step. In model 1, we considered 9 benchmark circuits (6 from ISCAS89 and 3 from open cores). We randomly split 50% of the total data into the training sample and the other 50% in the testing sample. In model 2, we use 6 benchmark circuit data for training purposes and use 3 benchmark circuit data for testing purposes which are unseen to the model. We utilize a regression neural network for predictions. To ensure robustness and reusability of the proposal, we validate our proposed models for two different technology nodes i.e. TSMC 65nm and TSMC 90nm. Experimental results show that the average mean square error for all the parameters for both the technologies is of the order of 10-3 while most of the parameter MSE is in the range of 10-5 to 10-7 for both the technology nodes. These data ensure robustness and re-usability of the proposal with a high level of accuracy.