Details
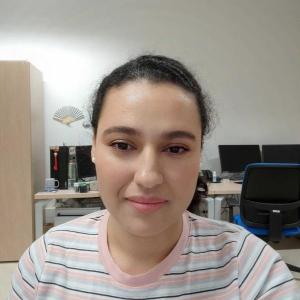
- Affiliation
-
AffiliationTelecom Paris
- Country
In order to overcome the limitations of traditional computer media, many researchers are turning to analog neural networks to get closer to the functioning of the brain. It is then necessary to use HW-friendly algorithms such as the Manhattan Update Rule (MUR) which is a version of the Back-Propagation (BP) algorithm compatible with the HW implementation. Although many studies use this algorithm for their hardware implementation of neural networks, no article proposes a study upstream of the latter. In this article, we propose an analysis methodology of the Manhattan algorithm allowing us to choose the weight update value ∆ω in order to obtain a minimum of 90% of accuracy. Also, we have answered the questions raised by the state of the art: we have therefore shown that it is possible to achieve the same performance as the BP in terms of precision (3.1% difference at max) and convergence speed. We have shown the link between the dependence of the number of epochs on the initialization of the weights, with the size of the network and the database. Finally, we gave indications for the choice of the version of the MUR (batch or stochastic) according to their speed of convergence.