Details
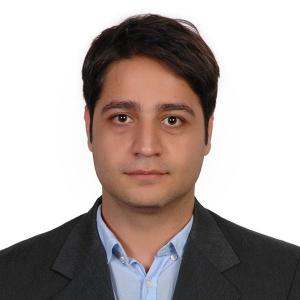
- Affiliation
-
AffiliationVirginia Polytechnic Institute and State University
- Country
This paper investigates direct and indirect learning methods to develop deep learning digital predistortion (DL-DPD)models and apply the models to improve the linearity of a power amplifier (PA). The two methods are applied to class-AB and class-F−1PAs designed with gallium nitride (GaN) on silicon carbide (SiC) high electron mobility transistors (HEMTs). The simulation results show that both direct and indirect DL-DPD methods improve the linearity of the class-AB PA by about 12dB and the class-F−1PA by 11 dB, while the indirect method offers marginally better performance. The paper shows the direct learning method leads to significant improvement of the DL-DPD method based on the memory polynomial. It also presents ad-vantages of a BiLSTM based on the neural network architecture to design direct/indirect DL-DPDs. Finally, it demonstrates that both direct and indirect methods can improve the linearity of class-AB and class-F−1 PAs without architectural changes.