Details
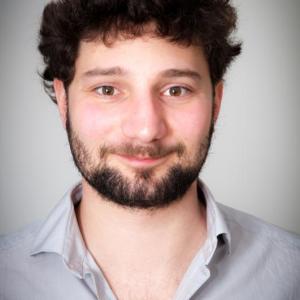
- Affiliation
-
AffiliationCEA-Leti
- Country
This paper reports the main State-Of-The-Art algorithmic enablers for compact Neural Network topology design. Embedding in-sensor intelligence to perform inference tasks generally requires a proper definition of a Neural Network architecture dedicated to specific purposes under Hardware limitations. Hardware design constraints known as power consumption, silicon surface, latency and maximum clock frequency cap available resources related to the topology, i.e., memory capacity and algorithmic complexity. We propose to categorize into 4 types the algorithmic enablers that force the hardware constraints as low as possible while keeping the accuracy as high as possible. First, Dimensionality Reduction (DR) is used to reduce memory needs thanks to predefined, hardware-coded patterns. Secondly, low-precision Quantization with Normalization (QN) can both simplify hardware components as well as limiting overall data storage. Thirdly, Connectivity Pruning (CP) involves an improvement against over-fitting while limiting needless computations. Finally, during the inference, a Dynamical Selective Execution (DSE) of topology parts can be performed to limit the activation of the entire topology.