Details
Presenter(s)
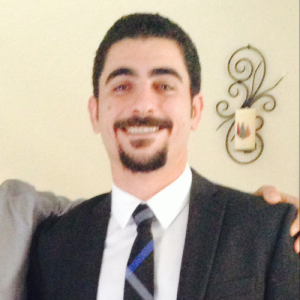
Display Name
Ismail Alkhouri
- Affiliation
-
AffiliationUniversity of Central Florida
- Country
Abstract
Existing work on adversarial attacks on classification tasks has focused on classifiers that make use of simple hypothesis testing models. In this work, we study the vulnerability of composite classifiers employing generalized likelihood ratio tests to adversarial perturbation attacks. We derive imperceptible adversarial attacks for a multiple composite hypothesis testing setting using gradient methods. The work considers scenarios where the attacker has access to the ground truth class. The classification performance, with and without perturbation, is characterized based on the notions of posterior sensitivity and specificity.