Details
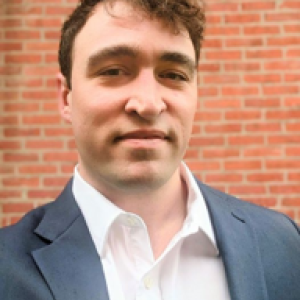
- Affiliation
-
AffiliationOhio State University
- Country
-
CountryUnited States
We propose a novel point-of-care (PoC) COVID-19 diagnostic system with ultrasound imaging. Our adaptive visual diagnostics utilize few-shot learning (FSL) to generate encoded disease state models that are stored and classified using a dictionary of known disease states. The novel vocabulary based feature processing of our pipeline adapts the knowledge of a pretrained deep neural network to compressed discrimative descriptions of the ultrasound images. The computational efficiency of our FSL approach enables high diagnostic deep learning performance in PoC settings, where training data is limited and the annotation process is not strictly controlled. We use the open source COVID-19 POCUS Dataset to validate our system\'s ability to distinguish COVID-19, pneumonia, and healthy disease states. The results of our empirical analyses demonstrate the appropriate efficiency and accuracy for scalable PoC use. The code for this work will be publicly available on GitHub upon acceptance.