Details
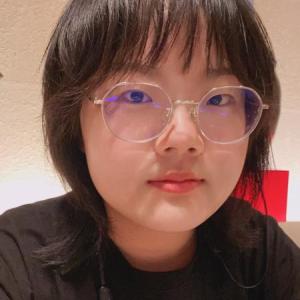
- Affiliation
-
AffiliationHosei University
- Country
The reconstruction of Compressed Sensing is iteration-based and contains numerous divisions, thereby costing tremendous processing time. In order to eliminate divisions, we adopt a sparse sensing matrix consisting mainly of zero-vectors. After deleting these zero-vectors, an invertible full-rank matrix is obtained. Then the iteration-based reconstruction procedure can be replaced by a matrix multiplication operated in one iteration. Moreover, because the inverse matrix is sparse and deterministic, the multiplication can be simply processed by the shift and add operators. The proposed architecture is verified on the Xilinx Artix-7 FPGA. The result shows that our work accelerates the state-of-the-art method by 65 times and achieves 81.92Gpixels/s reconstruction.