Details
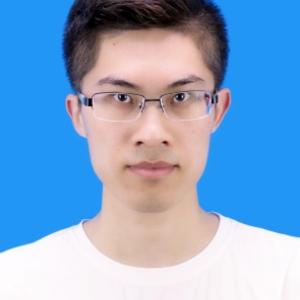
- Affiliation
-
AffiliationShanghaiTech University
- Country
-
CountryChina
Binarized convolutional neural network (BCNN) is a promising and efficient technique toward the landscape of Artificial Intelligence of Things (AIoT) applications. In-Memory Computing (IMC) is widely studied to accelerate the inference task of BCNN to maximize both the throughput and energy efficiency. However, existing IMC circuits and architectures are only optimized for a fixed kernel size and nominal voltage operation, which poses practical limitations on optimal network architecture exploration and additional energy efficiency benefits. In this paper, we propose a reconfigurable, near-threshold IMC-based BCNN accelerator. Our IMC design is scalable for different kernels sizes (3$\times$3$\times$d and 5$\times$5$\times$d) and achieves high resource utilization for both cases. A novel IMC bitcell is proposed for reliable near-threshold operation. Implemented in 55nm, our proposed IMC design achieves 5526 TOPS/W energy efficiency at 0.4V, which is 6.38$\times$ higher when compared to state-of-the-art designs.