Details
Presenter(s)
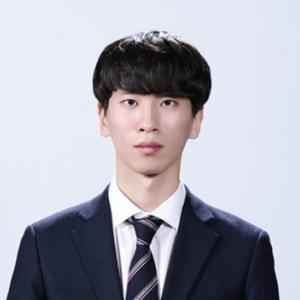
Display Name
Seokchan Song
- Affiliation
-
AffiliationKorea Advanced Institute of Science and Technology
- Country
Abstract
Low power real-time online learning object detection (OD) processor is proposed for mobile devices. First, novel quantization: multi-scale linear quantization (MSLQ) and MSLQ-aware PE are proposed. Second, channel-wise gradient skipping based on temporal correlation is proposed. These schemes reduce ~56% of computation and ~30% of EMA rather achieves improved accuracy. Gradient norm clipping with norm estimation achieves accuracy improvement with few additional computations. Proposed online learning OD processor is implemented in 28 nm CMOS and achieves 78 mAP of detection accuracy at YouTube-Objects dataset. It shows outstanding performance-49.5 mW power consumption and 34.4 fps-real-time online learning OD on mobile devices.