Details
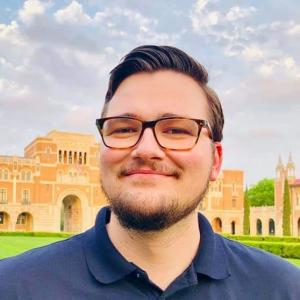
- Affiliation
-
AffiliationDuke University
- Country
-
CountryUnited States
Spike-timing-dependent plasticity is emerging as a simple and biologically-plausible approach to learning, and specialized digital implementations are readily available. Memristor technology has been embraced as a much denser solution than digital SRAM implementations of STDP synapses, with plasticity capabilities built into the physics of these devices. textit{One-selector-one-memristor} (1S1R) arrays using volatile memristor devices as selectors are capable of the desired synaptic behavior using efficient spike-events, but previous literature has only explored the dynamics of single 1S1R synapses, or a group of synapses for a single neuron. When placed in the context of an SNN, unintentional synapse disturbances are revealed that must be addressed. We present a technique for spike-timing-dependent plasticity learning, enabled for dense 1S1R technology and utilizing efficient single-spike encoding. This technique is leverages the arrays dynamics to produce models that are stable, resilient to noise, and power-efficient.