Details
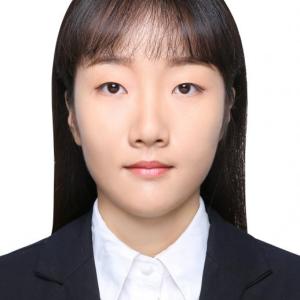
- Affiliation
-
AffiliationFudan University
- Country
-
CountryChina
This paper presents an ultra-low-power keyword spotting processor using an algorithm-architecture co-design approach. Joint MFCC-CNN ternary weight quantization is proposed to reduce power consumption. The Mel filter and the DCT module are merged into one matrix multiplication. The merged coefficients and the weights of the rest NN classifier are ternary-quantized, causing less than 3% accuracy loss but 39x energy efficiency improvement. Moreover, a Computing-on-Memory-Boundary macro is adopted to store the quantized coefficients and weights, and perform matrix multiplications. Compared to the existing computing-in-memory technology, the proposed technique can reduce power consumption due to the higher utilization ratio. To verify the proposed techniques, a keyword spotting processor prototype is designed with 28nm CMOS technology. Simulation results show that the prototype achieves power consumption of 11.6μW under a power supply of 0.72V and a clock frequency of 250KHz.