Details
Presenter(s)
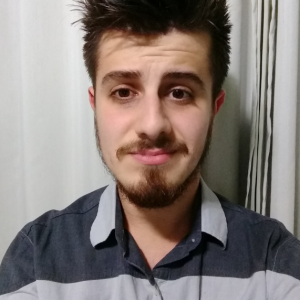
Display Name
Brunno Abreu
- Affiliation
-
AffiliationUniversidade Federal do Rio Grande do Sul
- Country
Abstract
The use of Machine Learning techniques in battery-powered devices has increased in recent years. Therefore, evaluating power-accuracy trade-offs of inference models has become important. This work explores decision trees architectures, analyzing the effects of model complexity and approximation in power and accuracy. By quantizing the inputs to limited widths, we increased the accuracy of the models in up to 8.7% compared to precise cases. In terms of power, the variation in model complexity was more significant than in input width, as we obtained reductions of up to 97% by decreasing the tree depth, compared to 88% when decreasing the width.