Details
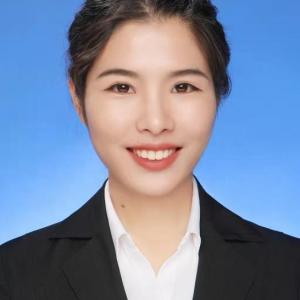
- Affiliation
-
AffiliationShanghai Jiao Tong University
- Country
Future brain-machine interface systems will require recording thousands of neural channels in parallel to acquire large-scale neuronal activity. High bandwidth action potential signal will overload the data communication bandwidth, and on-site spike sorting can extract essential information, but it requires extensive computational resources to achieve high classification accuracy. This demands for high hardware resources, especially in large-scale real-time sorting systems. Therefore, to reduce the hardware complexity without compromising the accuracy of spike sorting, a customized unsupervised training engine corporate with distributed and optimized sorting channels is presented. A mixed-domain feature set is extracted in each channel. Feature based sorting is performed. The alarm signal is constant computation and will request training engine intervention when needed. The proposed system is implemented in 180 nm CMOS process, consumes only 0.33 \\textmu W/channel when processing at 24 KHz and 1.8v and occupies 0.0023 mm$^2$/channel