Details
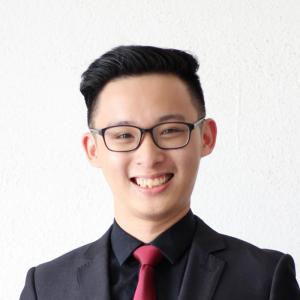
- Affiliation
-
AffiliationMultimedia University
- Country
-
CountryMalaysia
During the Coronavirus Disease 2019 (COVID-19) pandemic, many countries have introduced the social distancing policy in public areas to stop the spread of disease by maintaining a physical distance between people. This paper proposes an Artificial Intelligence (AI)-powered social distancing surveillance system that can detect pedestrians through video surveillance and monitor the social distance between them via Inverse Perspective Mapping (IPM) in real-time. The proposed system was deployed on the devices located at the network edge such as IoT devices and mobile devices to enable real-time response with low data transmission latency. To bypass the restriction on the computational and memory capacity for the edge devices, the proposed system was optimized through fixed-point quantization. From the evaluation results, the optimized models are almost 4 times smaller as compared to the original models. The best trade-off between speed and accuracy can be achieved with a 27.1% improvement in speed and 2% degradation in accuracy.