Details
Presenter(s)
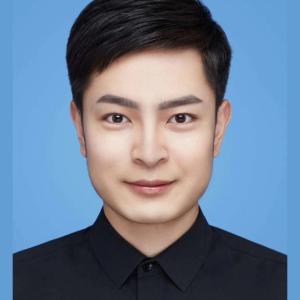
Display Name
Chao Zhang
- Affiliation
-
AffiliationTsinghua University
- Country
Abstract
This paper designs SaleNet - an end-to-end convolutional neural network (CNN) for sustained attention level evaluation using prefrontal electroencephalogram (EEG). A bias-driven pruning method is proposed together with group convolution, global average pooling (GAP), near-zero pruning, weight clustering and quantization for the model compression, achieving a total compression ratio of 183.11x. The compressed SaleNet obtains a state-of-the-art subject-independent sustained attention level classification accuracy of 84.2% on the recorded 6-subject EEG database in this work. The SaleNet is implemented on a Artix-7 FPGA with a competitive power consumption of 0.11 W and an energy-efficiency of 8.19 GOps/W.