Details
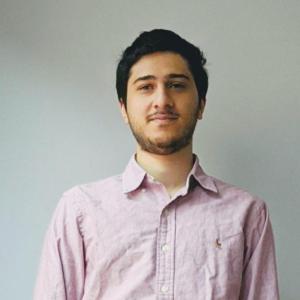
- Affiliation
-
AffiliationYork University
- Country
The design, optimization, and validation results of a patient-specific seizure detection algorithm are presented. The algorithm employs both mono-variate (spectral energy) and bivariate (narrow-band phase synchrony) features. The computational complexity of both feature extraction and brain state classification is optimized to enable the algorithm’s integration into a low-power implantable/wearable microprocessor. The patient specificity of the algorithm includes (a) the nonlinear RBF-SVM classifier's hyperplane characteristics, (b) band selection for phase extraction, and (c) channel selection (dimensionality reduction) for spectral energy extraction. The algorithm’s performance is validated on pre-recorded EEG data from 23 patients (969 hours, 198 seizures in total) and shows a seizure detection sensitivity and specificity of 96.87% and 99.95%, respectively. A comparison to the state of the art in terms of various design and performance parameters is presented.