Details
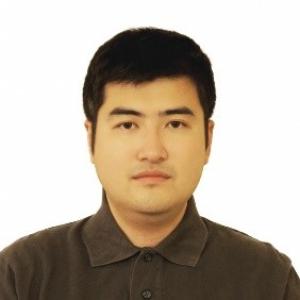
- Affiliation
-
AffiliationGeorgia Institute of Technology
- Country
In this work, we propose a mixed-precision RRAM-based compute-in-memory (CIM) architecture that supports on-chip training. In particular, we split the multi-bit weight into the most significant bits (MSBs) and the least significant bits (LSBs). The forward and backward propagations are performed with CIM transposable arrays for MSBs only, while the weight update is performed in regular memory arrays that store LSBs. Impact of ADC resolution on training accuracy is analyzed. We explore the training performance of CIFAR-10 dataset based on a VGG-like network using this Mixed-precision IN-memory Training architecture, namely MINT, showing that it can achieve ~91% accuracy under hardware constraints and ~4.46TOPS/W energy efficiency. Compared with the baseline CIM architectures based on RRAM, it can achieve 1.35× higher energy efficiency and only 31.9% chip size (~98.86 mm2 at 32 nm node).