Details
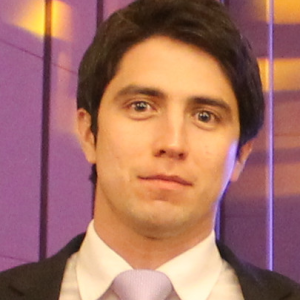
- Affiliation
-
AffiliationTechnical University of Dresden
- Country
In this paper, we architect, design, implement, and test a handcrafted, hardware Convolutional Neural Network, named BioCNN, optimized for EEG-based emotion detection and other similar bio-medical applications. The architecture of BioCNN is based on aggressive pipelining and hardware parallelism that maximizes resource re-use and minimizes memory footprint. The FEXD and DEAP datasets are used to test the BioCNN prototype that is implemented using the Digilent Atlys Board with a low-cost Spartan-6 FPGA. The experimental results show that BioCNN has a competitive energy efficiency of 11 GOps/W, a throughput of 1.65 GOps that is in line with the real-time specification of a wearable device, and a latency of less than 1 ms, which is much smaller than the 150 ms required for human interaction times. Its emotion inference accuracy is competitive with the top software-based emotion detectors.