Details
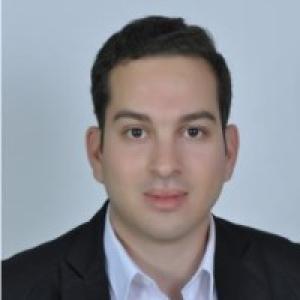
- Affiliation
-
AffiliationWayne State University
- Country
In this article, we generate a velocity profile of an autonomous car-generated path using the Frenet frame technique. The obtained data is used to be transferred into the cloud for update purposes, taking into consideration new traffic information, and sent back to the vehicle to adapt. The extreme variability of the data could be vulnerable to cyber-attacks and could alter the path planning process, by modifying the intended path or even block the transaction. We consider this exchange as a streaming service due to its high flux. After identifying the adequate cyber-attacks, we used a time-series approach to design our cyber-attacks Intrusion Detection System (IDS), which is a hybrid deep learning model using Long Short-Term Memory (LSTM) Autoencoder (AE). We compare the performance of our model, using specific metrics, with other models to put in evidence its adaptability with autonomous systems, as a time-series application, on the road. Our proposed model achieves a high accuracy of 98.7%, compared to other models.