Details
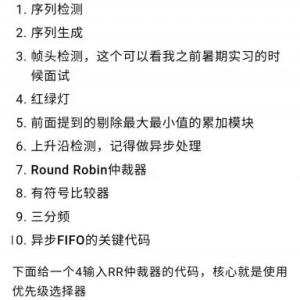
- Affiliation
-
AffiliationFudan University
- Country
Although the calibrator based on neural networks performs well in nonlinearity calibration for SAR-Pipelined ADC, it suffers from considerable hardware complexity. This paper proposes a network structure called layer-based neural network (LSNN) to optimize this calibrator. Compared with the traditional structures, LSNN has the following advantages. First, it optimizes storage and logic simultaneously. Second, it reduces the storage read and write bandwidth by half at least. Third, a simplified computing mechanism that reduces the adders for backpropagation by half can be introduced based on LSNN. A 14-bit 60 MS/s ADC fabricated in 28-nm process is used to verify the feasibility of the proposed structure. The measurements show that the SFDR and SNDR of the ADC calibrated by LSNN increase from 68.3 dB and 44.6 dB to 95.4 dB and 65.4 dB, respectively. Compared with the traditional neural network structures, the LSNN can optimize up to 76.7% of the logic area, 53.2% of the storage and 64.8% of the bandwidth.