Details
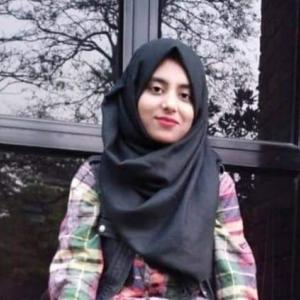
- Affiliation
-
AffiliationLUMS
- Country
Seizure type identification plays a pivotal part in the diagnosis and management of epileptic seizure disorder. Unfortunately, did not get much attention in past decades due to the unavailability of databases with seizure type marking. Seizure types not only assist the neurologist in deciding the correct drug and its dosage but precaution. This work proposes and implements an early diagnostic and management (EDM) system to assist the neurologist in type identification (5-classes) of the seizure activity at run time and also features an interactive graphical user interface (GUI). In the GUI, temporal, spectral (along with source localization) and spatial plots can be viewed along with the seizure data classified based on its types. The system utilizes a discrete wavelet transform (DWT) and k-nearest neighbor (KNN) based on feature extraction and classification. The system is validated using 31 patient’s recordings from Temple University Hospital (TUH) EEG Database. Our system achieves a 5-class classification accuracy, sensitivity and specificity of 97.7%, 92.9%, and 98.7%, respectively, for patient-wise cross-validation.