Details
Presenter(s)
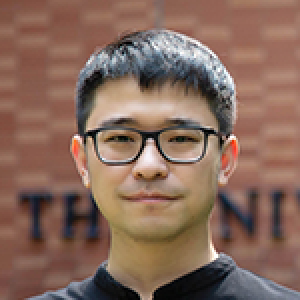
Display Name
Can Li
- Affiliation
-
AffiliationUniversity of HK
- Country
Abstract
There is an increasing amount of effort to build a fast, energy-efficient, large-scale, and, most importantly, reliable memristor crossbar system for in-memory analog computing. Silicon transistors are used as selectors and on-chip control peripheral circuits to fully unleash the power of the emerging devices. Still, unexpected computing errors occur because of non-ideal device performance. Herein, we review two promising solutions. The first one is the in-situ training of memristor neural networks to self-adapt various defects, and the second one is an analog error-correcting code that detects and corrects unexpected errors with minimum hardware overhead.