Details
Presenter(s)
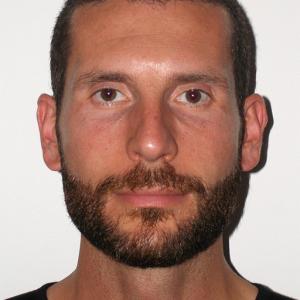
Display Name
Daniele Linaro
- Affiliation
-
AffiliationPolitecnico di Milano
- Country
Abstract
Load forecasting plays a crucial role in the operations of electric utilities and so several algorithms have been developed over the years to tackle this problem: most recent solutions use machine learning techniques to increase the granularity of the prediction. Here, we employ a framework based on long short-term memory networks to estimate the average power consumption of a single building equipped with solar panels. We show which measurements are more important for an accurate forecast and test several prediction horizons in order to find the best trade-off between training speed and prediction accuracy.