Details
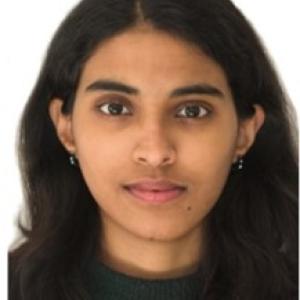
- Affiliation
-
AffiliationUniversity College Dublin
- Country
Quality assessment at the network edge aids in the elimination of corrupted data prior to storage or transmission. A k-Nearest Neighbor (k-NN) binary classifier is used for identifying whether the acquired electrocardiogram record is of acceptable quality for further processing and transmission. Feature vectors used for classification were derived from the raw signal using skewness and kurtosis-based Signal Quality Indicators for electrocardiogram. The proposed approach achieved a classification accuracy of 97.18 % with an estimated complexity that corresponds to 12.72 fJs Energy-Delay-product (EDP) in terms of multiplications used. To further reduce computational complexity and power consumption, an approximate multiplier was used, and this method was found to exhibit an accuracy of 96.48 %. The EDP, while using an approximate multiplier for classifying a single record was found to be 34.5 % lower at 8.333 fJs, and is within the power budget of a typical IoT device.