Details
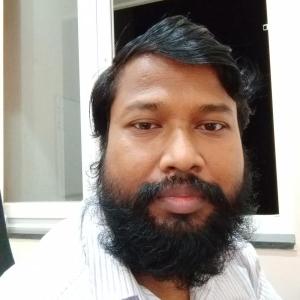
- Affiliation
-
AffiliationNational Institute of Technology Karnataka
- Country
An on-chip network has become a powerful platform for solving complex and large-scale computation problems in the present decade. However, the performance of bus-based architectures, including an increasing number of IP cores in systems-on-chip (SoCs), does not meet the requirements of lower latencies and higher bandwidth for many applications. A network-on-chip (NoC) has become a prevalent solution to overcome the limitations. Performance analysis of NoC's is essential for its architectural design. NoC simulators traditionally investigate performance despite they are slow with varying architectural sizes. This work proposes a machine learning-based framework that evaluates NoC performance quickly. The proposed framework uses the linear regression method to predict different performance metrics by learning the trained dataset speedily and accurately. Varying architectural parameters conduct thorough experiments on a set of mesh NoCs. A highlight of the experiments includes the network latency, hop count, maximum switch, and channel power consumption as 30-80 cycles, 2-11, 25µW, and 240µW, respectively. Further, the proposed framework achieves accuracy up to 94% and a speedup of up to 2228x.