Details
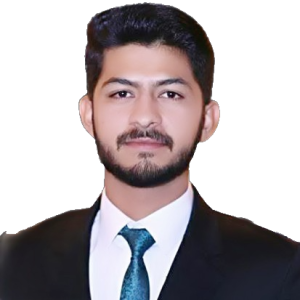
- Affiliation
-
AffiliationLahore University of Management Sciences
- Country
Blood pressure (BP) is considered one of the key vital signs that provide valuable medical information about the cardiovascular activity. Conventionally, cuff-based devices are used to measure BP which limits their usage for continuous monitoring. This paper presents a cuff-less BP estimation processor using photoplethysmography (PPG) signals with a Deep Neural Network (DNN). Spectral and temporal features are extracted from the PPG signals and then used to train and evaluate the machine learning (ML) algorithms. The proposed algorithm is evaluated using the MIMIC II database for systolic blood pressure (SBP) and diastolic blood pressure (SBP) estimation. The proposed BP estimation processor is implemented using a 180nm CMOS process with an area of 3.45mm2 and consumes 73µW. It achieves a mean absolute error in systolic BP of 0.0657±4.7 mmHg and diastolic BP of -0.792±4.61 mmHg which outperforms the state-of-the-art BP estimation algorithms.