Details
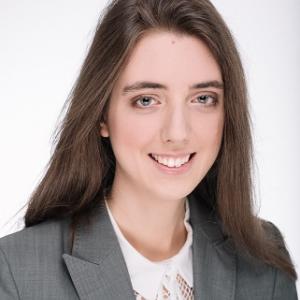
- Affiliation
-
AffiliationUniversité Catholique de Louvain / ICTEAM Institute
- Country
While bottom-up neuromorphic designs are ideal as experimentation platforms, their key challenge lies in demonstrating an efficiency advantage for real-world scenarios. In order to leverage the data sparsity of spike-based neuromorphic retinas for adaptive edge computing, we follow a top-down approach and propose SPOON, a 28-nm event-driven CNN. It embeds online learning with the biologically-plausible direct random target projection (DRTP) algorithm. With 313nJ/classification at 0.6V and a 0.32-mm² area, accuracies of 95.3% (on-chip training) and 97.5% (off-chip training) on MNIST demonstrate that SPOON reaches the efficiency of conventional machine learning accelerators. We demonstrate on-chip learning for event-based sensors with N-MNIST.