Details
Poster
Presenter(s)
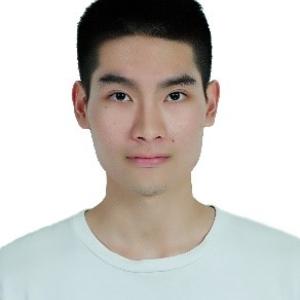
Display Name
Hong-Han Lien
- Affiliation
-
AffiliationNational Chiao Tung University
- Country
Abstract
In this paper, we integrate the proposed IF-based Batch Normalization into the binary weight spiking neural network to reduce the hardware cost. Our model achieve 90.28% accuracy on CIFAR-10 using only 8 time steps. In addition, the proposed reconfigurable vectorwise accelerator can handle the different models at will, and supports the multi-bit input encoding layer and layer fusion mechanism according to the configuration. Our design can operate at 25.9 TOPS/W under peak efficiency with better power and area efficiency than the previous reconfigurable design and higher flexibility than the fixed network design.