Details
Poster
Presenter(s)
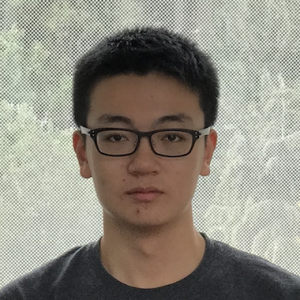
Display Name
Jian Meng
- Affiliation
-
AffiliationArizona State University
- Country
Abstract
The high computational complexity and a large number of parameters of deep neural networks become the most intensive burden of deep learning hardware design. The resistive RAM (RRAM) crossbar based in-memory computing has recently emerged as a promising DNN acceleration technique. To fully exploit crossbar-based IMC efficiency, a systematic software and hardware design for compression are necessary. This paper presents a system-level design considering the low precision quantization, structured pruning, and RRAM crossbar mapping. Both hardware and software results of the proposed design show the large performance improvements and superiority compared with the recent state-of-art compression design.