Details
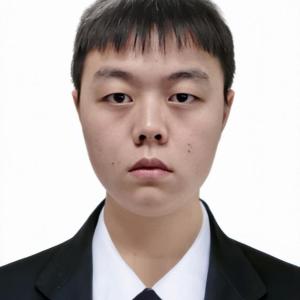
- Affiliation
-
AffiliationSouthwest University of Science and Technology
- Country
-
CountryChina
Knowledge distillation is an extensively researched model compression technology, which uses a large teacher network to transmit information to a small student network. The key point of the knowledge distillation method to improve the performance of the student network is to find an effective method to extract the information from the feature. The attention mechanism is a widely used feature processing method to process feature effectively and obtain more expressive information. In this paper, we propose to use the dual attention mechanism in knowledge distillation to improve the performance of student networks, which extracts information from the spatial and channel dimensions of the feature. The channel dimension attention will search `what' channel is more meaningful, and the spatial dimension attention will determine `where' part of the feature is more expressive in a feature map. We have conducted extensive experiments on different datasets shown that by implementing a dual attention mechanism to extract more expressive information for knowledge transfer, the student network can achieve performance beyond the teacher network.