Details
Poster
Presenter(s)
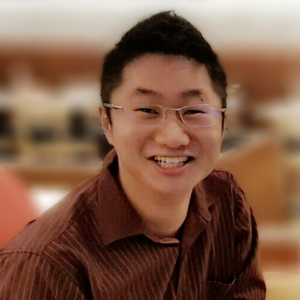
Display Name
David Wong
- Affiliation
- Country
Abstract
Wearable Artificial Intelligence-of-Things (AIoT) devices demand smart gadgets that are both resource and energy-efficient. In this paper, we explore efficient implementation of binary convolutional neural network employing function merging and block reuse techniques. The hardware implemented in field programmable gate array (FPGA) platform can classify ventricular beat in electrocardiogram achieving accuracy of 97.5%, sensitivity of 85.7%, specificity of 99.0%, precision of 92.3%, and F1-score of 88.9% while consuming only 10.5-µW of dynamic power dissipation.