Details
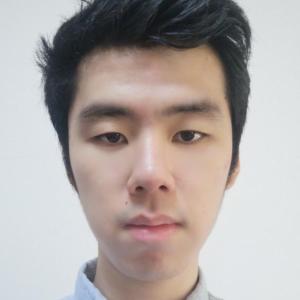
- Affiliation
-
AffiliationNanyang Technological University
- Country
The increased latency and power consumption due to data movement between memory and ALU have become the major obstacle in modern big-data and machine learning applications. Beyond von-Neumann architectures, particularly in-memory computing, is under intensive research to overcome this memory access bottleneck. In this work, we propose a 2T2R ReRAM structure that supports ternary content addressable memory (TCAM), logic in-memory operations, and in-memory dot product for Deep Neural Networks (DNNs) besides the normal non-volatile memory (NVM) functionality. This is achieved by employing reconfigurable sense amplifiers and novel word-line drivers. The proposed architecture can serve as a high-density storage system as well as an accelerator for data-intensive applications. Simulation results verify that the proposed 2T2R structure functions correctly for TCAM search, logic in-memory operations and in-memory dot product.