Details
Poster
Presenter(s)
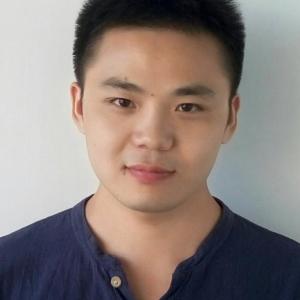
Display Name
Chenglong Zou
- Affiliation
-
AffiliationPeking University
- Country
Abstract
In this paper, we propose a adjustable quantized training algorithm for artificial neural networks (ANNs), and provide two (temporally or spatially) rate-based conversion methods for spiking neural networks (SNNs), both of which can be easily mapped to specific neuromorphic platforms. Besides, this novel method can be generalized to various network architectures and adapted to dynamic quantization demand. Experimental results on MNIST and CIFAR-10 datasets demonstrate that the proposed deep spiking neural networks yield the state-of-the-art classification accuracy and need much less operations compared with their ANN counterparts. Our source code will be available upon request for the academic purpose.