Details
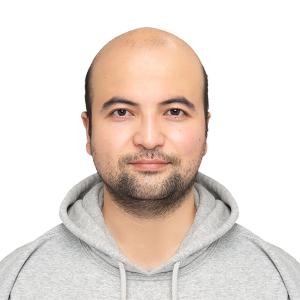
- Affiliation
-
AffiliationChungbuk National University
- Country
-
CountrySouth Korea
In this paper, we propose a novel decentralized channel resource allocation algorithm for V2V communication based on deep multi-agent reinforcement learning (MARL). Each vehicle as an independent agent uses its local observation to select the optimal transport block (TB) from pre-configured resource pool (environment). We apply an actor-critic learning method which allows each agent to have centralized training and decentralized execution. In centralized training, all agents share their local observations and actions in the critic networks. Therefore, each agent can estimate the policies of other agents during decision-making process. In decentralized execution, each agent uses its local observation to optimize local policy independently. Each action taken by the agent in actor network will be judged by its private critic network