Details
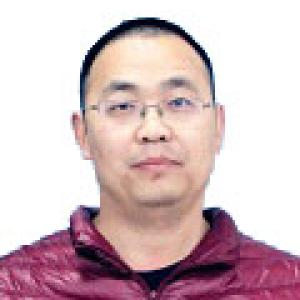
- Affiliation
-
AffiliationHunan University of Science and Technology
- Country
Deep learning-based video inpainting can fill the missing or undesired regions with spatial-temporal consistent contents without obvious visually distortion. Although the original purpose of deep inpainting is to repair flawed videos, it can also be adopted for malicious purpose, e.g., removal of specific objects. Therefore, automatically locating the inpainted regions is a challenging task in video forensics. This paper proposes a new forensic refinement framework to localize the deep inpainted regions by considering the spatial-temporal viewpoint. Firstly, we design a spatiotemporal convolution to suppress redundant for highlighting deep inpainting traces. Then, a detection module is constructed with four concatenated ResNet blocks, and two upsampling layers to achieve a rough location map. Finally, a modified U-net based refinement module is developed for the pixel-wise localization map. Deep inpaiting video datasets created by the state-of-the-art deep inpainting method, have been evaluated, and extensive experimental results clearly demonstrate the efficacy of the proposed approach.