Details
Poster
Presenter(s)
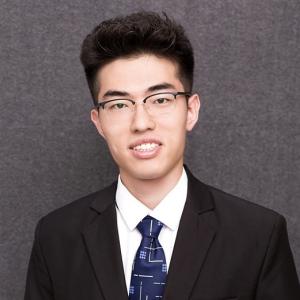
Display Name
Jiachen Xu
- Affiliation
-
AffiliationCarnegie Mellon University
- Country
Abstract
Machine-learning-based readout channels are presented for direct data symbol detection via decision-tree classification with gradient boosting for multiple-actuator data storage systems. The proposed learning module integrates energy-efficient linear classifiers to extract features and structures from raw readback signals. The results demonstrate high detection accuracy, which is robust to inter-symbol interference (ISI) and jitter noise. The low-complexity machine learning module classifies raw data with an accuracy rate higher than 95.8% in real-time and consumes only 53 mW.