Details
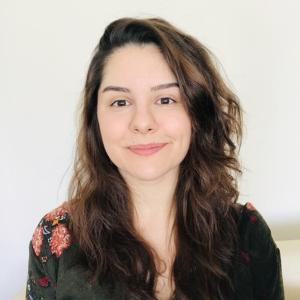
- Affiliation
-
AffiliationTélécom Paris
- Country
In the existing works of analog implementation of Equilibrium Propagation, the impacts of the learning rate, alpha (α), and the scaling factor of the feedback current, beta (β), have not been discussed. This work analyzes the impacts of the scaling factor of feedback current and the learning rate together with the ratio of those two parameters on the algorithm convergence. An Equilibrium Propagation circuit has been implemented on Cadence Virtuoso for a simple task to test the impacts of alpha (α) and beta (β) parameters. Numerical simulations are carried out in a Python-Spectre interface that we implemented. Detecting the optimum ranges for alpha (α) and beta (β) values is particularly influential on the algorithm performance. Our simulation results show that the algorithm only converges for distinctive alpha (α) and beta (β) values.