Details
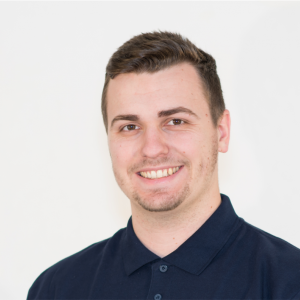
- Affiliation
-
AffiliationFraunhofer Institute for Microelectronic Circuits and Systems
- Country
Emerging smart sensor systems are the main driver of innovation in many fields of application. A prominent example is condition-based monitoring and especially its subdomain fault diagnosis. The integration of advanced machine and deep learning-based signal processing into sensor systems enables new intelligent condition monitoring solutions. However, the data-based nature of machine and deep learning methods still impedes their applicability in many cases, due to a severe lack of data. In this paper, we introduce a new hybrid physics- and data-based framework aiming to solve the issue of small datasets for vibration-based fault diagnosis applied to rolling-element bearings. The framework combines a vibration simulation model and a neural network with embedded physics-based knowledge into a physics-guided neural network. Our approach aims to generate physically consistent data for the training of fault classifiers without extensive data acquisition.