Details
Poster
Presenter(s)
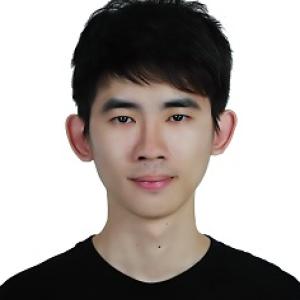
Display Name
Jheng-Yi Chang
- Affiliation
-
AffiliationNational Tsing Hua University
- Country
Abstract
In this thesis, we proposed a high utilization energy-aware real-time inference DCNN accelerator designed to tackle three important issues, hardware utilization, external memory access and computation complexity. The hardware implementation of the proposed accelerator architecture under the TSMC 40 nm technology reaches 1.152 Tops/s with 554.57 mW total power in 3.59 mm^2 area size. The energy efficiency of the proposed accelerator reaches 2.08 Tops/W.