Details
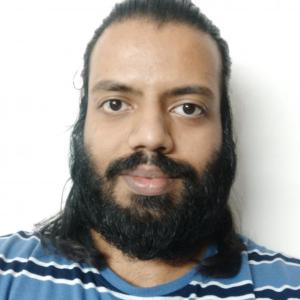
- Affiliation
-
AffiliationIndian Institute of Technology, Hyderabad
- Country
The recent advancement in semiconductor and computing technology has empowered the field of structural health monitoring in many ways. This work introduces a deep learning-based architecture, ‘FCNet,’ to predict acoustic emission signals arising from deformations like corrosion and fatigue crack. The suggested model uses a lightweight framework that takes advantage of the convolutional neural networks to demonstrate the implicit ability of feature identification, which removes the time-consuming stages of feature selection and extraction. The model’s performance was proved using a dataset of 8566 corrosion and fatigue AE signals. To identify corrosion and fatigue AE signals, the model attained a 99.78 percent accuracy, demonstrating the efficacy of the suggested model for real-time reliability. The importance of this research for the industry is that it will provide a lethal approach for identifying metal deformation causes and, as a result, reducing accidents